![]() |
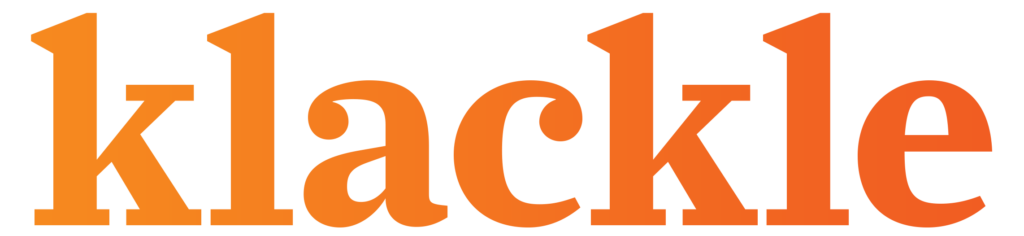
Experience the power of your data at work.
Compete with facts, not assumptions.
Artificial intelligence is no longer science fiction. It’s changing the way the world works. Right here, right now. Is your business ready for the speed, scope, and scale of the next industrial revolution? Klackle is a Toronto-based machine learning and advanced analytics consulting firm that can help you solve big problems with big data.
What we do isn’t magic. It’s math.
Don’t risk your business on a blackbox.
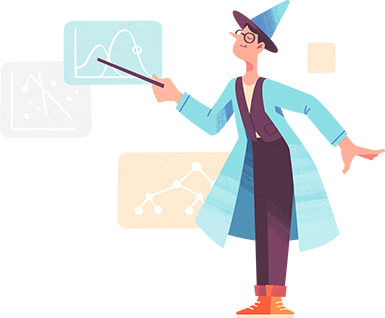
Our experience at Klackle is clear: Treating machine learning like a blackbox limits organizational acceptance. Lasting impact demands analytics that inspire confidence. We take a collaborative approach to ensure not just the best answer, but an answer that makes sense for everyone involved. We aim to deliver not just a solution to your machine learning problem, but a way to solve the next problem too. That means understanding a solution, not just getting one.
Big data deserves big expertise.
Strategy and data, all at once.
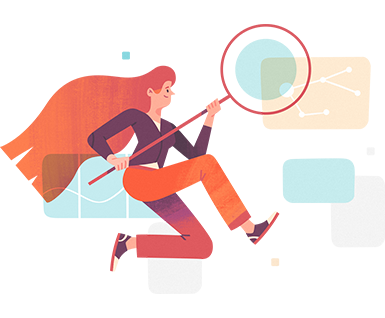
Data science isn’t just about the numbers. It’s about the business. Our team’s experience combines classical statistics, machine learning, and management consulting. We’ve worked at top consulting firms and graduated with advanced degrees from top academic programs. That’s how we get measurable results, not theoretical ones. That’s why Klackle is trusted by enterprises and start-ups alike. That’s why we view data science through a strategic lens.
Our work speaks for itself.
The proof is in the numbers.
Klackle’s collaborative, research-driven advanced analytics approach has helped our team solve business-critical analytics problems across industries and functions. Retail. Automotive. Financial Services. Telecommunications. Consumer Packaged Goods. Durables. Manufacturing. Data science consulting is versatile. We are too. Everything from pricing, to forecasting, to inventory optimization. Here are a few recent examples of our statistical consulting work.
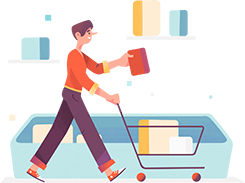
A large retailer sought to algorithmically optimize pricing for its catalogue of over 100K products. Combining historical point-of-sale data with user experiments, we estimated the price elasticity for all products in inventory. We then designed an algorithm to automatically update pricing based on actual and predicted competitor and consumer behaviour. Our project increased net margin by 5% while improving consumer price perception.
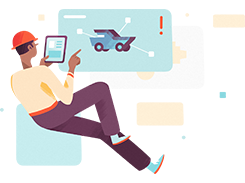
An industrial enterprise client sought to determine the number of redundant pieces of long-lead-time equipment to purchase to minimize downtime risk while also minimizing cost. We built a predictive algorithm to estimate when equipment would fail, and then simulated potential outcomes to understand the probability of simultaneous failures. Our work quantified the risk-minimization value of the client’s $20M investment.
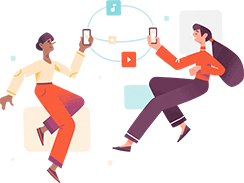
A new social media smartphone app start-up sought to measure the similarity between users to provide better recommendations. The sparsity of their data resulted in the poor performance of off-the-shelf algorithms. Our project yielded a novel recommendation algorithm approach to improve quality and increase user engagement. The smartphone app is known for the quality of its recommendations.
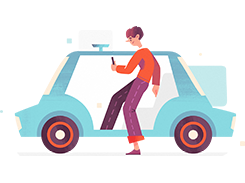
A client sought perspective on the growth potential of the ridesharing market. Using publicly-available sources, we built a model that predicted whether one would use ride sharing in the next thirty days with 98% accuracy. Combining our work with geospatial analysis of census data, a database of ridesharing receipts, financial statements, and growth trends, we estimated ridesharing usage in the US at the zip-code level.